How foresight complements business-planning
- Stephen Plimmer
- Apr 9, 2023
- 8 min read
Updated: Apr 18, 2023
In this post, a foresight study is proposed to be a natural complement to a business planning process and forecast. It helps to surface risks that merit investment, and fuel innovative product or marketing ideas.
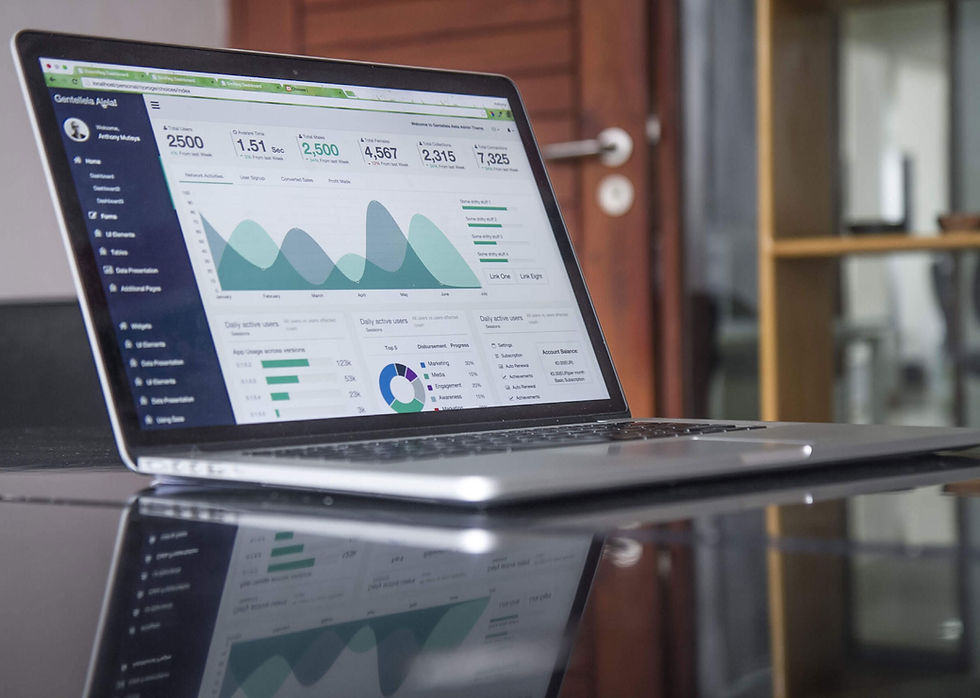
Forecasts
People working in many roles spend a lot of their working lives predicting the future.
Each year, finance teams forecast the year ahead and write an associated rationale in the form of a business plan.
Marketers predict how well different possible campaigns will perform, to choose the combination with the most likely chance of success.
In Operations, Supply-chain and Service teams, managers might spend considerable time predicting how many staff are going to be required to meet demand over the coming days, weeks and months.
Now and again, functions might consider implementing new technologies or processes to make improvements, and predict the impact on the customer experience - thus revenue and retention rates - and cost.
Businesses and key departments are thereby continuously and routinely making “forecasts” where the time horizon can vary from a day to several years. However, for all of these problems, the methodology is very usually a variation on a theme: using historical performance trends as the basis for assuming what will happen in the future: Take, for example, three approaches to forecast future sales, applying different levels of complexity:
Basic: The most basic forecasts are simply calculated by extrapolating past sales trends and making an informed guesstimate about how that trend might continue, but without considering the underlying drivers too hard. Whatever was driving the sales numbers in the past is often assumed to carry on driving sales numbers, perhaps to a bit of a lesser or greater extent. A nuanced version of this would be to carry out the same analysis on the individual line-items in the sales forecast (e.g. by different products, or customers-segments) and then add up them up,
Driver-analysis: A more nuanced approach might involve considering how the drivers of those outcomes, likes sales revenue, are changing and building a business model: For instance, the business might assume it will spend more money on different marketing campaigns in coming years, improve the return-on-sales by improving campaign performance over time through learning, while also adding a new channel to market that it assumes will deliver a further contribution to sales, and derive the future sales forecast from the result of these assumptions.
Market-based: A rarer approach to forecasts would to employ some sort of market model, such as a product lifecycle curve, and make a forecast based on that. Rather than assuming that external product-market environment is unchanging, which the two methods above effectively do, such a model accounts for the fact that all product-markets grow, plateau and decline. The forecaster would consider where the product life cycle has reached for the company's different products, and so what the trend is likely to do next; assuming the company’s sales trends will be correlated in some way. Market-, as well as sales-, data is needed to take such an approach.
The key assumption of forecasts
While not often explicitly stated, there an inherent assumption from making forecasts in the ways above i.e. that the future is actually predictable. The attitude is: We might not know what the future will be exactly, but there is a defined future world we are heading to, we can have a good go at calculating what it will be, and end up close enough to plan our business around it. There is a second assumption that the past is a good indicator of the future.
On the one hand, these assumptions have some basis: The Survey of Annual Earnings Forecasts by Refintiv has been conducted since the 1970s in different guises, and typically shows that analysts can predict company earnings one year out within 10-15%. A further study in the Journal of Business Forecasting that examined the sales forecasts of 13 global companies returned a 13% average deviation from the actual sales numbers.
A 2015 paper by Jana Ries and colleagues examined the accuracy of sales numbers over a ten year period in the notoriously-unpredictable technology sector. Even there, the accuracy of forecasts for company level sales figures were 69% and product line sales were 78%.
However, the problem comes when forecasts are for longer time horizons, as found to be the case over the past 2-3 decades in a number of studies e.g. Armstrong (2001) and Sanders and Mandrot (2003). The consistent conclusion is that forecast accuracy falls as the time-horizon lengthens.
Even amongst the world's best so-called “Superforecasters” - an elite group of forecasters found from intensive multi-year competitions run in the US and involving tens of thousands of people - this relationship was found to hold, particularly when forecast horizons extended beyond 1-2 years. (Tetlock, P. E., & Gardner, D., 2015)
Quite simply, as time moves forward, the number of complex, unforeseen factors on a business accrue. The business environment (market, political, social, cultural, environmental et al) also evolves and so to predict a business performance accurately would require the impossible feat of accurately predicting all of these changing environmental drivers, and their impacts.
In more recent decades, the pace of technological innovation has increased incredibly compared to the rate of new inventions through history. New technology performance, that could not have been predicted even a decade ago, impact business models and thereby the way entire industries “do things”
Such complexities and their unforeseeable consequences are the reason for a number of famous, incredibly inaccurate predictions from very bright people that have littered the past century:. In 1948, educator Mary Somerville predicted “Television won’t last. Its a flash in the pan.” In 2004, Bill Gates prophesied that "Two years from now, spam will be solved.". In 2007, Steve Balmer prophesied, “"There's no chance that the iPhone is going to get any significant market share." These people saw the present situation, and they saw some or many of the factors at play, but not the interplay of factors that ultimately derailed their vision.
Separately, discrete shock events (e.g. COVID, the Ukraine War) happen.
Moreover, even when a trend might be predicted, like "people will communicate more through computers" at the end of the 1990s, it's far harder to predict if it “goes viral”, because it sets off a self-perpetuating chain of events that no-one in the chain might have foreseen, like the growth of Facebook from 2004.
The other significant contributor to forecast inaccuracies comes from human biases, where foreseeable factors are overlooked or misinterpreted. For instance, “confirmation bias” tends to cause people to seek out information that validates their pre-existing beliefs, rather than challenge them. Human beings tend to display an ‘overconfidence’ bias in forecasts, which can be inadvertently supported by aspects of an organisation's culture, such as expectations to provide a positive growth “story”. The “end of history illusion” describes how people believe that things won’t change as much in the upcoming decade, say, as they did in the last. (See our separate article on “How foresight studies help overcome organisational biases about their future”),
So, this begs the question of asking, “if a business can’t predict the future, how is it supposed to plan for it?” This is where the discipline of foresight becomes useful.
Foresight studies
Whereas forecasts start from “here and now” and look forward, seeking to estimate the single most accurate picture of the future, foresight studies entertain the possibility that there are multiple possible futures for the world and, therefore, for the business within those worlds. Foresight studies embrace the idea, fully, that we simply cannot say how the future will pan out: there are a range of futures.
Foresight studies don’t aim to predict the future, but rather, by exploring possibilities, offer the benefit of better preparing for it.
However, while there is an element of creativity and speculation involved with creating a scenario of a future business in a future market, foresight studies are nonetheless still built on data and evidence. However, it tends to be a different type of data and evidence than the oft-quantitative historic data used for forecasts. It typically requires more sources.
For instance, foresight studies typically use a combination of i) trends (industry, business, technology, social etc), ii) lessons from how markets, companies and customers have behaved in the past that won’t likely change even if the situations do (e.g. people reduce the effort to do a task if they can), iii) the trajectory of current and newer technologies and their prices and performance, and iv) the new ideas, imagery, language and technologies that are just starting to emerge in the peripheries of society, science and business; particularly places from which new ideas and trends are known to emerge.
The end product of a forecasting exercise is a trend line, showing sales numbers. The end product of a foresight or trends study is an inventory of opportunities, threats and potential strategic and commercial options for the business - along with some appreciation of scenarios when such options, or similar options, would become applicable.
To be forewarned is to be forearmed.
Forecasts and foresight-studies: Complementary, not competing
Therefore, a foresight study is not an alternative, competing way of generating a sales forecast. Rather a foresight study and a business forecast are complementary exercises. A foresight study explores the reasons that the sales forecast will be wrong, particularly as the time horizon expands.
Practically, the nature of foresight studies varies by the scope of the landscape under consideration and the time horizon.
Concerned about the future survival of the planet, and dealing with existential questions such as “how will the world survive and prosper if the population shrinks?” (as it is expected to do later in this century), supranational bodies like the World Economic Forum concern themselves with scenarios that are 50+ years away.
.
Closer to home, and relevant in the moment, commentators have wrestled with the question of what work will look like after AI replaces or augments human work, as some expect it could to significant and different degrees over coming decades. In his 2017 book, “WTF: What's the Future and Why Its Up to Us”, Tim O’ Reilly dedicated a chapter to contemplating the scenario that “we don’t have to run out of jobs.”
For a smaller to medium sized business, the potential opportunities and threats created by AI might indeed be one of the factors that are keeping people awake at night. However, arguably, so should other 3-10 year “question marks”, like meeting the new demands of Gen-Z consumers, paying energy costs that might be higher by an unknown amount, and addressing higher expectations to support staff mental-wellbeing and face legal claims for not doing. Meanwhile scenarios for global trade could still take different turns and impact supply chains.
By the time such trends start to impact the business, it will likely be too late to either exploit or mitigate them effectively or even at all.
This offers a very practical way that traditional business planning and foresight exercises can co-exist: the latter providing the basis of sensitivity analysis and content for broader risk management and innovation/growth strategies.
More broadly, foresight studies can help to create robust mid- and longer-term strategies, inform risk management, fuel product and innovation pipelines, and create the motivation to explore new markets or collaborations.
Ultimately, while forecasting is a necessity to keep the cogs of business turning, foresight exercises can tell you what the nature of the cogs might be in the future that the business will need, or even whether the future machine might contain cogs at all.
Sources
Refinitive I/B/E/S estimates https://www.refinitiv.com/en/financial-data/company-data/ibes-estimates
Lawrence, M., & O'Connor, M. (2010). Sales forecasting accuracy: The impact of forecasting methods and the characteristics of the product. Journal of Business Forecasting, 29(4), 4-12.
Jana Ries and colleagues, Björn C. Schäfer, and Florian von Wangenheim, "Forecasting sales of new and existing products by category captains: Empirical investigation in the technology industry"
Armstrong, J. S. (2001). Evaluating forecasting methods. International Journal of Forecasting, 17(3), 399-417.
Sanders, N. R., & Manrodt, K. B. (2003). Forecasting accuracy: A comparison of traditional methods and neural networks in the case of purchase order forecasts. International Journal of Production Economics, 84(2), 165-178.
Tetlock, P. E., & Gardner, D. (2015). Superforecasting: The Art and Science of Prediction. Crown Publishers.
Opmerkingen